Workshop Details
Goal
Recent advances in machine learning saw the rise of powerful automatic methods to build robust predictive models from data. To enhance understanding and improve performance, human-centred approaches have been pursued. In interactive visual machine learning (IVML) systems such as [1,2,3,4,5], a human operator and machine collaborate to achieve a task, mediated by an interactive visual interface. Typically, an IVML system comprises an automated service, a user interface, and a learning component. In IVML, the role of the human operator may be not only to interpret and understand the underlying models or decisions, but to also actively act on, and react to these models. This brings forth first the known problems of intelligibility, trust, and usability issues, but also many open questions with respect to the evaluation of the various facets of the IVML system, both as separate components, and as a holistic entity that includes both human and machine intelligence. Identifying the best evaluation methods to follow for validating machine learning (ML) and interactive visual machine learning (IVML) models remains a challenging topic.
The goal of the EVIVA-ML workshop is to bring together visualization researchers and practitioners to discuss experiences and viewpoints on how to effectively evaluate interactive visual machine learning systems. Ultimately, the workshop aims to form a plan to develop a research agenda for IVML evaluation.
- S. Amershi, J. Fogarty, D. Weld. Regroup: Interactive machine learning for on-demand group creation in social networks. SIGCHI Conference on Human Factors in Computing Systems. ACM, 2012.
- E. T. Brown, J. Liu, C. E. Brodley, R. Chang. Dis-function: Learning distance functions interactively. Visual Analytics Science and Technology (VAST). IEEE, 2012.
- W. Cancino, N. Boukhelifa, E. Lutton. Evographdice: Interactive evolution for visual analytics. Evolutionary Computation (CEC). IEEE, 2012.
- M. El-Assady, R. Sevastjanova, F. Sperrle, D. Keim, C. Collins. Progressive learning of topic modeling parameters: a visual analytics framework. Transactions on Visualization and Computer Graphics. IEEE, 2018.
- H. Kim, J. Choo, H. Park, A. Endert. Interaxis: Steering scatterplot axes via observation-level interaction. Transactions on Visualization and Computer Graphics. IEEE, 2016.
Topics
The workshop aims to foster discussion on topics related to the evaluation of interactive visual machine learning systems, including but not limited to:
- User studies of existing or novel IVML systems
- Computational and automatic evaluation of IVML systems
- Comparative studies of variants of IVML systems
- Case studies to evaluate the usability of IVML systems
- Surveys on evaluation methods for IVML
- Novel evaluation methods for IVML
- Applications of existing evaluation methods for IVML
- Heuristic and other low-cost approaches for evaluating IVML
- Evaluation metrics for IVML (e.g., integrating model and user metrics)
- Taxonomies of tasks for IVML
- Lessons learnt and reflections on evaluation methods for IVML
Submissions
We invite short paper submissions (research or position papers) varying between two to four pages (excluding references). Submissions will be reviewed by the organizing committee and selected external reviewers, and will be chosen according to relevance, quality, and likelihood that they will stimulate and contribute to the discussion.
Submissions must be formatted according to the VGTC conference style template.
Papers are to be submitted online through the Precision Conference System (VIS 2019 Workshop on EVIVA-ML track).
Accepted contributions will be made available electronically as a collection of preprints. Authors will retain copyright.
Keynote
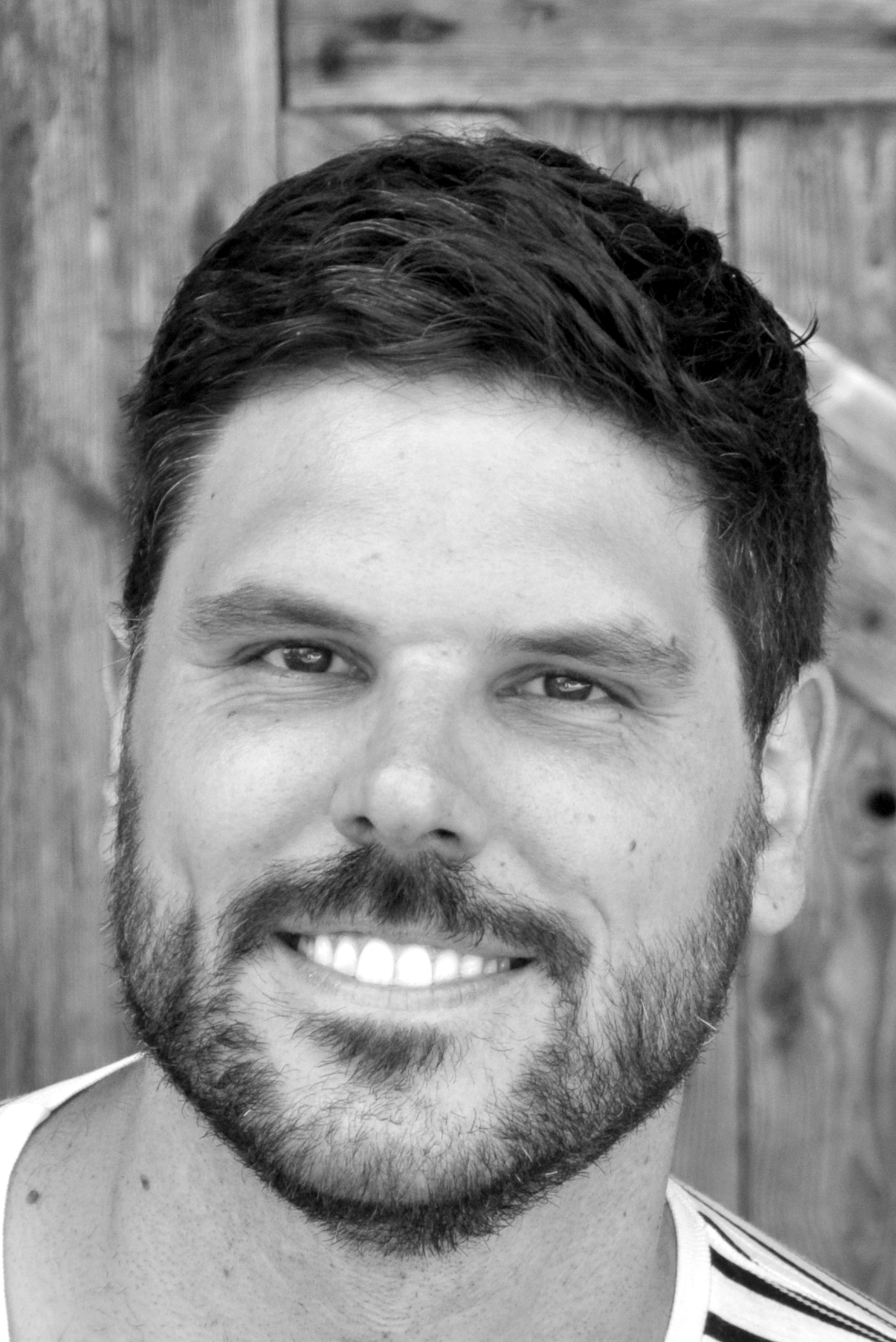
Title
Vis, ML, Eval: Experiences from the Trenches
Abstract
Over the last years, the work at the intersection of Machine Learning (ML) and Visualization (Vis) has increased notably. I will view this intersection from two angles. In *Vis for ML* the main idea is that visualization can help machine learning researchers and users to gain interesting insights into their models and data. It includes the flourishing fields of interactive machine learning and explainable AI. However, there is also a growing interest in using *ML for Vis*, which bears the potential to automatize parts of the visualization design process. Interestingly both areas are closely tied to human-subject evaluation, but in different ways. In the talk, I will report on our experiences working in Vis for ML, ML for Vis, and their evaluation.
Biography
Michael Sedlmair is a junior professor at the University of Stuttgart, where he works at the intersection of human-computer interaction, visualization, and data analysis. His specific research interests focus on information visualization, interactive machine learning, virtual and augmented reality, as well as the research and evaluation methodologies underlying them.
Programme
Organizing Committee
- Nadia Boukhelifa (INRA, FR)
- Anastasia Bezerianos (Univ. Paris-Sud and INRIA, FR)
- Enrico Bertini (NYU Tandon School of Engineering, USA)
- Christopher Collins (Uni. of Ontario Institute of Technology, CA)
- Steven Drucker (Microsoft Research, USA)
- Alex Endert (Georgia Tech, USA)
- Jessica Hullman (Northwestern University, USA)
- Michael Sedlmair (University of Stuttgart, DE)
Advisory Committee
- Remco Chang (Tufts University, USA)
- Chris North (Virginia Tech, USA)